In the same way we would with humans: through education. This should not surprise anyone, as many of the ideas that are AI generated are a reflection of how we understand ourselves.
When comparing the creation of AI with human values, several interesting analogies can be drawn.
Education and learning: Just as we educate children with values and social norms, we train AI with data that reflects our principles. If the data is biased or incomplete, AI will learn imperfectly, much like a person can develop prejudices if they grow up in a biased environment.
Culture and context: AI, like humans, do not develop in isolation. They absorb the ideas, biases, and norms of the societies that create them. Therefore, the biases and values integrated into AI reflect the social and cultural values present in the data with which it is trained.
Morality and ethics: Just as humans need ethical and moral systems to make decisions, AI needs guidelines to avoid harmful or incorrect decisions. For example, AI systems may require encoded "ethical principles," similar to how humans develop codes of ethics and morality.
Perception of fairness: Just as humans constantly redefine what we consider fair or unjust as society progresses, AI also needs to be adjusted to ensure that its decisions are equitable and just, according to our evolving standards.
These analogies show that the creation of AI is the sum of technical process and human values and ethics.
At the last DreamForce event, one of the talks that caught my attention the most was an interview with Charles Oppenheimer, the grandson of the renowned physicist immortalized in the Oscar-winning movie. This grandson, who worked at Salesforce years ago, emphasized the relevance of this historical moment, marked by the widespread adoption of AI. He made an interesting parallel with the discovery of atomic energy at the end of World War II that led to the Cold War as a result of society's mismanagement. However, now we have the opportunity to learn from history and do better.
Salesforce places great importance on the ethical and human aspects of AI implementation, both on its platform and throughout its ecosystem. In fact, this is significant, and we can see it clearly: in the AI Associate certification, 39% (the highest percentage) of the exam focuses on this aspect.
In one of its Trailhead modules, Salesforce states: “As AI becomes increasingly integrated into our lives, we must address the issue of bias and fairness in AI systems. The importance of ensuring fairness and upholding ethical guidelines cannot be ignored. Biases, discrimination, and ethical dilemmas arise when AI systems are not developed and used responsibly.”
But what is bias?
Bias refers to the presence of systematic and unfair distinctions or preferences that can produce discriminatory outcomes. Recognizing bias is the first step in addressing and mitigating its impact. Once bias is identified, developers, data scientists, and business users can take corrective actions. Bias can arise from systematic errors or social prejudices. These can infiltrate an AI system in several ways, such as incorrect measurement or imbalanced representation in the data.
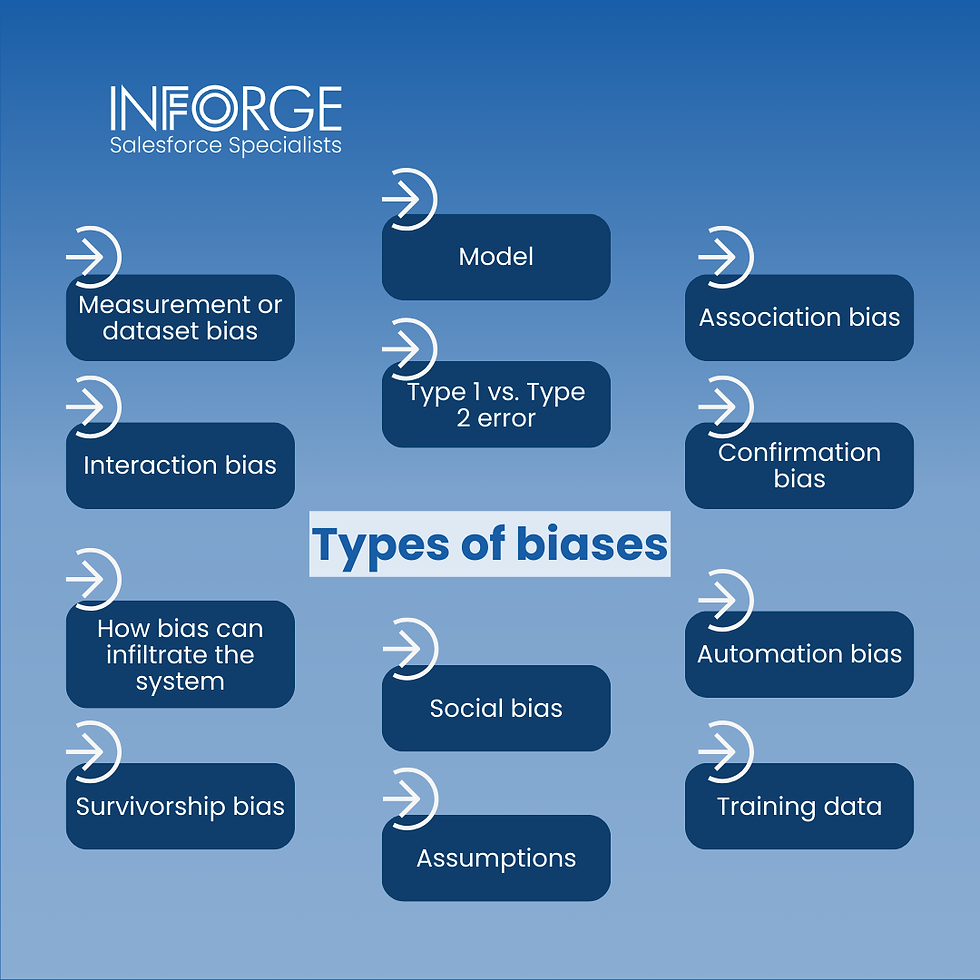
According to Salesforce, these are the types of biases we should be alert to:
Measurement or dataset bias: Occurs when data is labeled or represented incorrectly or oversimplified, leading to erroneous outcomes. An example is a system misclassifying a white dog as a cat due to a bias in the training data.
Type 1 vs. Type 2 error: Type 1 error is a false positive, while Type 2 error is a false negative. Both types of errors can affect a model's accuracy, such as in the granting of loans by a bank.
Association bias: This bias manifests when data is labeled according to stereotypes. An example is assigning toys to boys or girls based on traditional gender roles.
Confirmation bias: Reinforces preconceived ideas. For example, a recommendation system may promote products similar to those a customer has already bought, reinforcing established patterns.
Automation bias: The system's values and limitations can influence outcomes. For instance, an AI-based beauty contest might discriminate by race due to a bias in the training data.
Social bias: Reflects historical prejudices and affects marginalized groups. The example of "redlining" shows how certain data, such as postal codes, can perpetuate racial discrimination in financial decisions.
Survivorship bias: Focuses only on successful outcomes, ignoring those that did not pass the process. This can lead to biased decisions in processes like employee hiring.
Interaction bias: Arises when humans intentionally influence the behavior of an AI system, such as teaching a chatbot to swear.
How bias can infiltrate the system: Bias can enter through developers, training data, or the social context in which the AI is used.
Assumptions: AI creators make assumptions about the data and how the system should function. Diverse teams and stakeholder inclusion can help limit bias.
Training data: If training data comes from a limited source, the model may learn and reinforce biased patterns, such as hiring candidates from a homogeneous group.
Model: The features used to train the model, such as race or gender, can cause bias. Some characteristics, such as names, can act as proxies for these.
Human intervention (or lack thereof): Modifying or not modifying training data can impact bias. Users should have the opportunity to provide feedback on the system’s recommendations to correct potential biases.
To generate effective AI, we must provide it with the best possible quality of data. But quality is not synonymous with quantity; quality means representativeness and diversity. It’s not enough to have large volumes of information; the data must reflect all facets of humanity to avoid perpetuating biases and inequalities. In this sense, AI should not only help us optimize processes but also make us more aware of our limitations and biases as a society. Perhaps, in the process of improving our systems, AI will force us to take a deep introspective look and ask whether we are truly building a more just and equitable future for everyone.
What if, instead of being the end of humanity as many predict, AI helps us take a closer look at ourselves?
Kommentare